For my Master's Thesis, I developed a new model using the concepts of Spatial Networks to use in predicting the future growth of the Tesla Supercharger network. That work and paper can be found here.
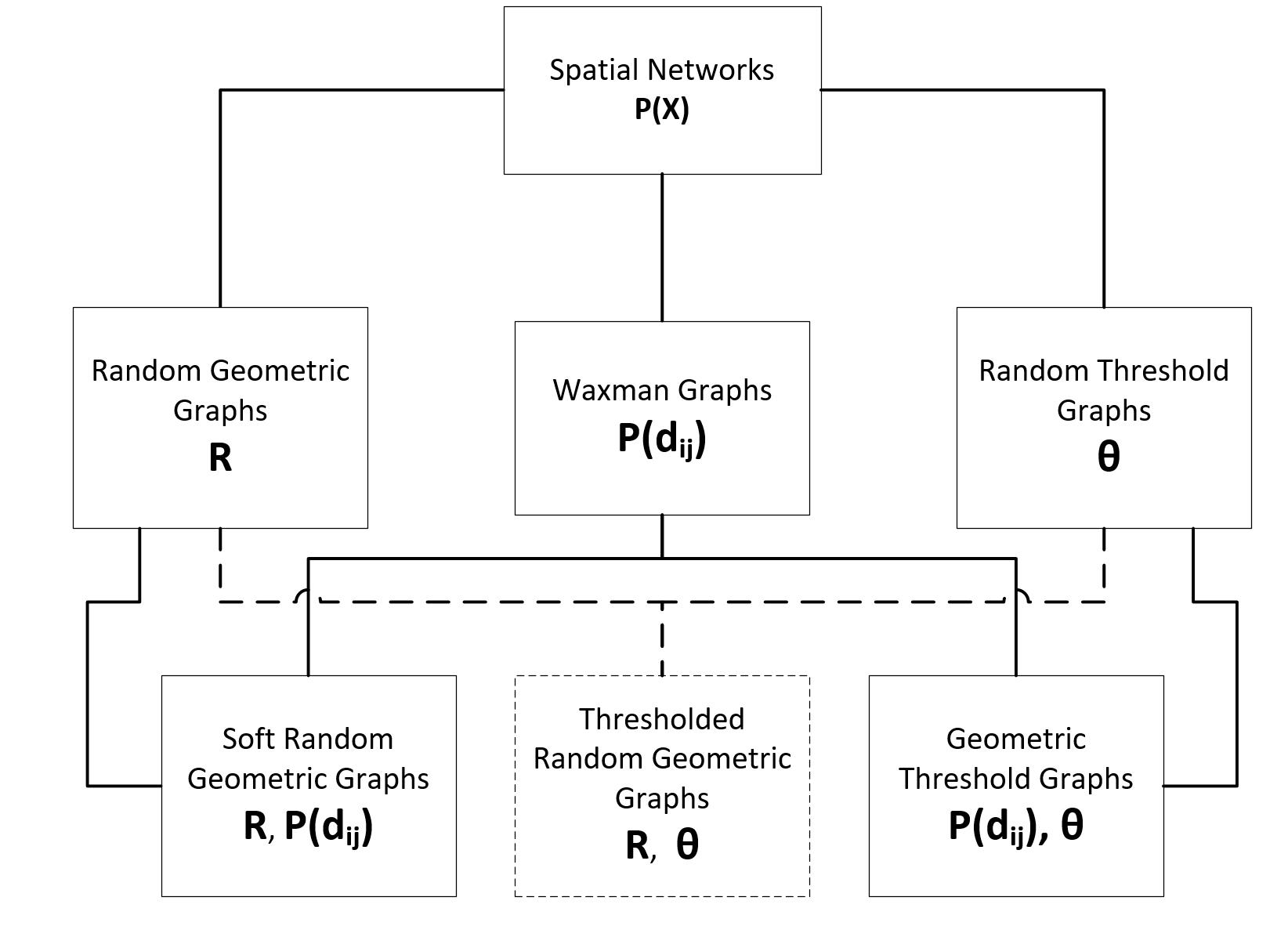
MAI-IML Exercise 4: Adaboost from Scratch and Predicting Customer Churn
Abstract
In this work, we develop a custom adaboost classifier compatible with the sklearn package and test it on a dataset from a telecommunication company requiring the correct classification of custumers likely to "churn", or quit their services, for use in developing investment plans to retain these high risk customers.
Adaboost from Scratch
Defined below is an sklearn compatable estimator utilizing the adaboost algorithm to perform binary classification. The input parameters for this estimator is the number of weak learners (which are decision tree stubs on a single, randomly selected feature) to train and aggregate to produce the final classifier. An optional weight distribution can also be passed to the classifier, which defaults to uniform if not set. This custom estimator will later be utilized to develop a classifier capable of predicting customer churn from labelled customer data.
Continue Reading...
Identifying Fraud from Enron Emails
Objective
Using the Enron email corpus data to extract and engineer model features, we will attempt to develop a classifier able to identify a "Person of Interest" (PoI) that may have been involved or had an impact on the fraud that occured within the Enron scandal. A list of known PoI has been hand generated from this USATODAY article by the friendly folks at Udacity, who define a PoI as individuals who were indicted, reached a settlement or plea deal with the government, or testified in exchange for prosecution immunity. We will use these PoI labels with the Enron email corpus data to develop the classifier.
Data Structure
The dataset used in this analysis was generated by Udacity and is a dictionary with each person's name in the dataset being the key to each data dictionary. The data dictionaries have the following features:
...
Continue Reading...
Data Munging and Analyzing Barcelona OSM Data
The XML data for the city boundary of Barcelona was downloaded from OSM to clean and transform into a json encodable structure to allow loading into MongoDB, providing storage and artbitrary querying to enable further data analysis of the Barcelona OSM dataset. I chose the Barcelona dataset because I just recently spent a few weeks there and noticed some pretty cool urban planning features of the city (ie. Avinguda Diagonal) that could be cool to explore further.
Inspired by the "Diagonality" of Barcelona streets and to give the scope of the project a little more focus, I will be attempting to analyze the Barcelona OSM data and see if I can generate a measure for the "degree of diagionality" for Barcelona and possibly compare this measure with other cities. Not knowing ahead of time the difficulty of this, I may eventually have to...
Continue Reading...