MAI-IML Exercise 4: Adaboost from Scratch and Predicting Customer Churn
Abstract
In this work, we develop a custom adaboost classifier compatible with the sklearn package and test it on a dataset from a telecommunication company requiring the correct classification of custumers likely to "churn", or quit their services, for use in developing investment plans to retain these high risk customers.
Adaboost from Scratch
Defined below is an sklearn compatable estimator utilizing the adaboost algorithm to perform binary classification. The input parameters for this estimator is the number of weak learners (which are decision tree stubs on a single, randomly selected feature) to train and aggregate to produce the final classifier. An optional weight distribution can also be passed to the classifier, which defaults to uniform if not set. This custom estimator will later be utilized to develop a classifier capable of predicting customer churn from labelled customer data.
Continue Reading...
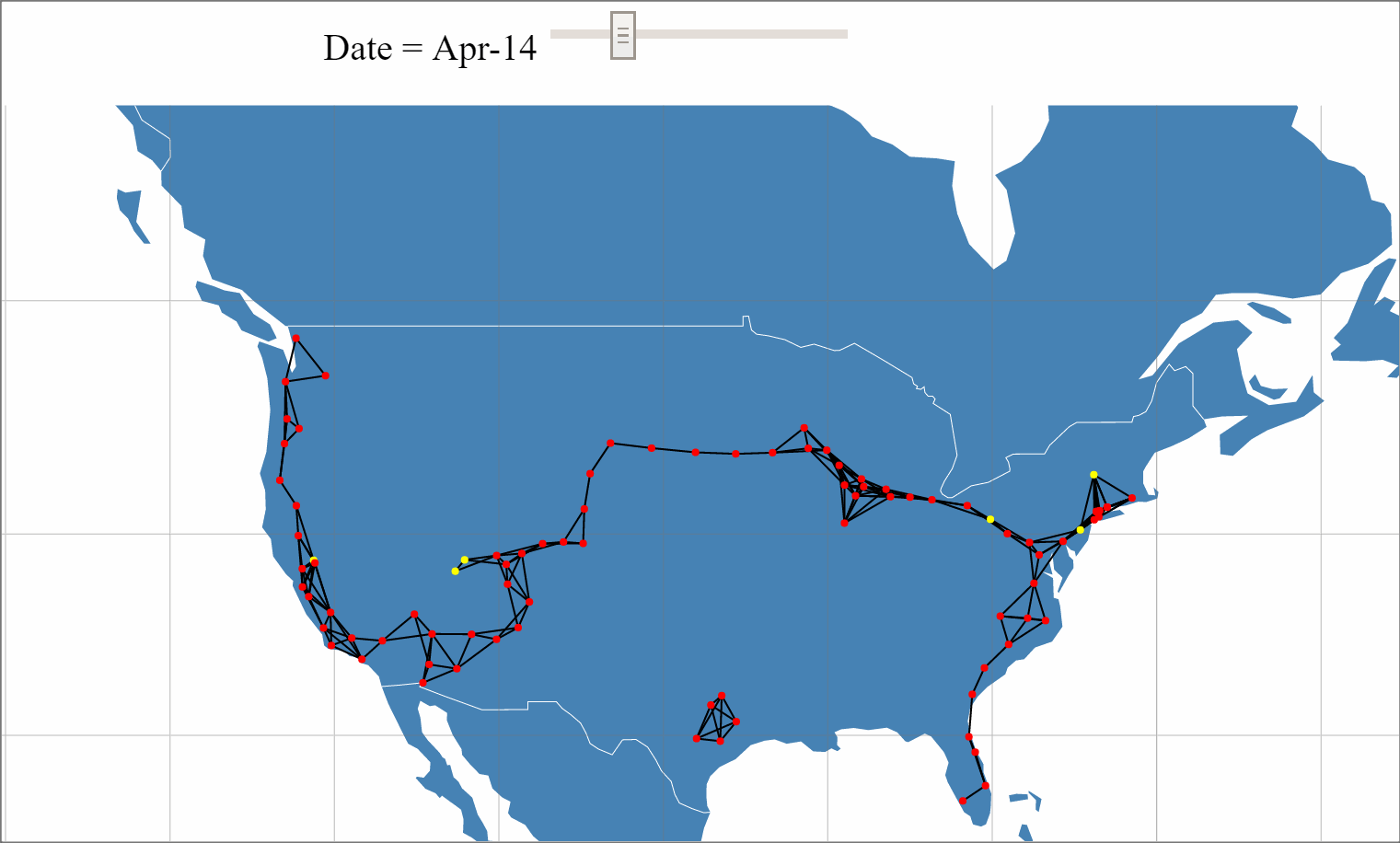
Introduction
With the recent unveiling of Tesla's Model 3 and pre-orders approaching 400,000, the internet has been buzzing with Tesla discussions and analysis. One of Tesla's key differentiators from other mass market Electric Vehicals (EVs) is its Super Charger (SC) network that provides 170 miles of range in 30 minutes source. With Elon Musk stating plans to double the size of the SC network by the end of 2017, a large amount of planning, resources and investment are being allocated to this network expansion.
An analysis to build a predictive mode for Tesla's Supercharger network expansion was performed using beaker notebooks.
The full analysis can be viewed here.
The interactive network data visualization can be viewed here
Visualization can be viewed here
Summary
This visualization attempts to encode the growth in size and scope of the scientific boundary for multiple disciplines by using the count of published scientific papers to the Arxiv pre-publishing website as a proxy for the "size" of a given scientific boundary. Meta data is collected using Arxiv's API. The titles of each paper in a given discipline and for a given year are passed into a "Bag of Words" categorization model that sorts each paper based on its abstract into a category, or defines a new category if a new cluster in the model emerges as more paper titles are added as the years progress. Each new category that is created is "born" from a parent category, which is determined as being the existing category that is most-like the newly created category, based on the words in the labels of each...
Continue Reading...
Identifying Fraud from Enron Emails
Objective
Using the Enron email corpus data to extract and engineer model features, we will attempt to develop a classifier able to identify a "Person of Interest" (PoI) that may have been involved or had an impact on the fraud that occured within the Enron scandal. A list of known PoI has been hand generated from this USATODAY article by the friendly folks at Udacity, who define a PoI as individuals who were indicted, reached a settlement or plea deal with the government, or testified in exchange for prosecution immunity. We will use these PoI labels with the Enron email corpus data to develop the classifier.
Data Structure
The dataset used in this analysis was generated by Udacity and is a dictionary with each person's name in the dataset being the key to each data dictionary. The data dictionaries have the following features:
...
Continue Reading...